RNA sequencing, also known as RNA-Seq, is an exceptionally potent and all-encompassing approach to examining the transcriptome of cells. The process of RNA-Seq revolves around constructing a library of complementary DNA (cDNA) for sequencing. To begin library construction, cellular RNA is first isolated, followed by quality control assessments to ascertain RNA integrity. Afterward, the RNA of interest in the library can be enriched through removal or screening techniques, and subsequently, it undergoes reverse transcription into cDNA prior to sequencing.
RNA-Seq finds extensive applications, ranging from fundamental explorations of cellular structure and function to the identification and analysis of various disease conditions in clinical samples. This technology enables both qualitative and quantitative detection of numerous RNAs in biological samples at specific time points. For instance, alterations in gene expression before and after a therapeutic intervention can be compared to determine the presence or absence of a disease. Moreover, RNA-Seq can identify selective splicing patterns, post-transcriptional modifications, and exon-intron boundaries. The acquired data holds invaluable insights into underlying cellular mechanisms, genome structure, disease-causing effects, and much more.
Total RNA Sequencing
Total RNA-Seq, also known as whole transcriptome sequencing, is a comprehensive method that encompasses the sequencing of all RNA molecules, both coding and non-coding. In this approach, RNA is sequenced after the removal of ribosomal RNA (rRNA), resulting in a diverse collection of RNA molecules. The initial isolation of total RNA yields a mixture of various RNAs, such as rRNAs, precursor messenger RNAs (pre-mRNAs), messenger RNAs (mRNAs), and different types of non-coding RNAs (ncRNAs), including transfer RNAs (tRNAs), microRNAs (miRNAs), and long fragments of non-coding RNAs (lncRNAs) that are not translated into proteins (transcripts longer than 200 nucleotides).
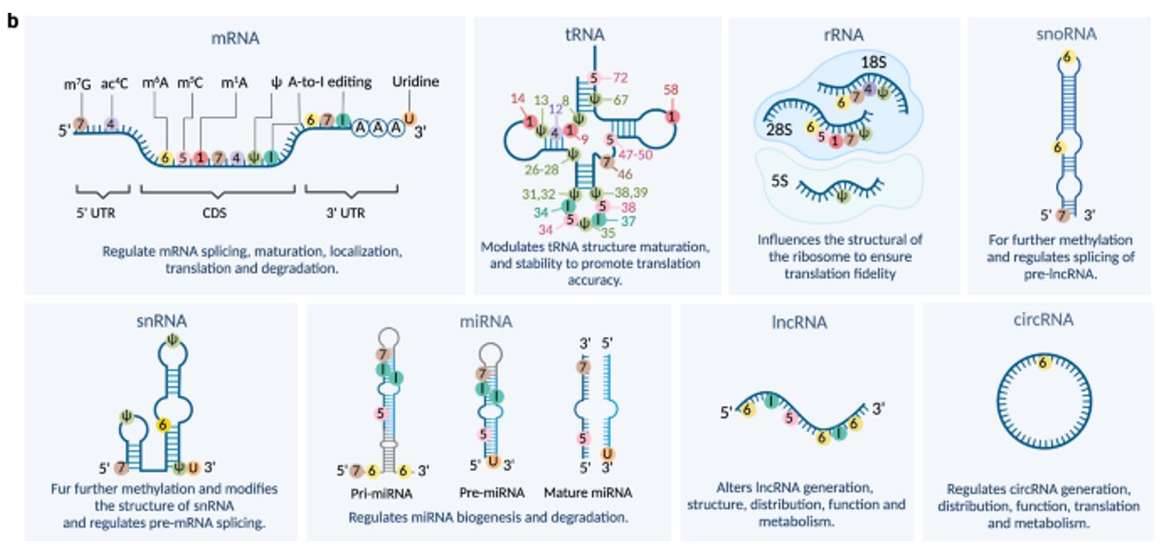
Different RNA subtypes. (Cui et al., 2022)
Total RNA-Seq offers insights into both coding RNAs and non-coding RNAs (e.g., lncRNAs and miRNAs), providing valuable information about regulatory regions, overall transcript expression levels, splicing patterns, and the identification of exons, introns, and their boundaries.
During sequencing, many lncRNA reads may overlap with mRNAs. It is noteworthy that approximately 20% of genes in the human genome are transcribed from opposite strands, leading to overlapping regions. Consequently, strand-specific total RNA sequencing methods are necessary to distinguish between these strands. Strand-specific sequencing techniques enable the identification of the specific DNA strand (coding or template strand) that generates the RNA transcript. Furthermore, they enhance the accuracy of gene expression data by improving the annotation of sequencing reads and facilitating the addition of matchable sequencing reads.
To enhance the sensitivity of sequencing, rRNA, which constitutes 80-90% of total RNA, is typically removed through debiasing. Eliminating rRNA transcripts allows for a greater concentration of sequencing reads on the desired transcripts, thereby increasing the sensitivity of the sequencing process. The removal of rRNA is particularly crucial when the expression level of the target transcript is low.
mRNA Sequencing
mRNA-Seq is the preferred choice when focusing on the coding region of eukaryotic targets. This method employs a screening technique to enrich for poly(adenylated) (poly(A)) RNAs. Since mRNAs constitute only a small fraction of the total RNA, sequencing only the mRNAs proves to be the most efficient and cost-effective approach if it aligns with the experimental objective.
In contrast to the rRNA removal step in total RNA-Seq, mRNA-Seq relies on Poly(A) affinity screening to enrich for mRNA. Both methods effectively eliminate rRNA from samples. The decision between rRNA removal and Poly(A) enrichment depends on various factors, including sample size and type (e.g., prokaryotes, animals, and plants), with each requiring different methods.
Sample enrichment or removal methods, such as ribosome removal or mRNA enrichment, enhance the quality of sequencing data in both Total RNA-Seq and mRNA-Seq workflows. These approaches enable sequencing of target RNA molecules while minimizing the waste of sequencing reads.
It is crucial to identify the desired information from the RNA-Seq data, as this helps exclude other types of RNAs from the sequencing process. If the focus is on the coding region, mRNA-Seq is the appropriate choice. Concentrating on mRNAs provides superior gene expression data since they constitute only 3-7% of the mammalian transcriptome. Compared to total RNA-Seq, mRNA-Seq allows for library preparation using smaller sample sizes while increasing sequencing depth.

mRNA-seq flowchart and data analysis pipeline. (Zhao et al. 2016)
Budget considerations are also important in selecting the appropriate method. Total RNA-Seq requires more sequencing data (typically 100-200 million sequencing reads per sample) and incurs higher costs compared to mRNA-Seq. If only mRNA information is required, mRNA-Seq offers greater sequencing depth and lower costs than total RNA-Seq. This is because sequencing reads (typically 25-50 million sequencing reads per sample) are concentrated on Poly(A)-enriched RNA molecules. For samples with limited starting material, mRNA-Seq is the most suitable method, providing better sequencing read data, lower costs, and requiring less starting material.
How to Choose mRNA Sequencing and Total RNA Sequencing
Choosing between total RNA-Seq and mRNA-Seq techniques requires careful consideration of the overall experimental objective, potential biological issues, and technical limitations. Each method offers unique advantages and disadvantages. Total RNA-Seq provides the most comprehensive transcriptome analysis by capturing all RNA species present in the sample, including non-coding RNAs and alternative splicing variants. On the other hand, mRNA-Seq focuses specifically on protein-coding transcripts, providing superior data on the coding regions of genes.
In addition to the experimental objective, several other factors should be taken into account. The sample type plays a crucial role in selecting the appropriate method. For samples with limited starting material, such as small tissue samples or single cells, mRNA-Seq is often preferred due to its higher sensitivity and ability to work with low input amounts. Total RNA-Seq, however, is more versatile and can handle a broader range of sample types, including degraded or partially fragmented RNA.
The sample starting volume should also be considered. If the available starting material is limited, mRNA-Seq may be a more viable option. Conversely, if a larger volume of starting material is available, total RNA-Seq can be performed more efficiently.
Project budget is another important factor. mRNA-Seq is generally more cost-effective since it focuses on a smaller portion of the transcriptome, whereas total RNA-Seq covers the entire transcriptome and may require a larger budget.
Furthermore, it is crucial to evaluate the technical limitations and requirements of each method. mRNA-Seq involves additional steps for mRNA enrichment, which can introduce biases. On the other hand, total RNA-Seq captures the entire RNA population but may have lower specificity for mRNA. Consider the available expertise and resources in laboratory for performing the chosen method.
By considering the experimental objective, potential biological issues, technical limitations, sample type, sample starting volume, and project budget, you can make an informed decision on whether to use total RNA-Seq or mRNA-Seq for your specific research needs. It is also advisable to seek advice from experts or bioinformatics professionals to ensure the most appropriate method is chosen.
References:
- Cui, Lian, et al. "RNA modifications: importance in immune cell biology and related diseases." Signal transduction and targeted therapy 7.1 (2022): 334.
- Zhao, Shanrong, et al. "Bioinformatics for RNA-seq data analysis." Bioinformatics—Updated Features and Applications: InTech (2016): 125-49.